Convergence of AI, ML and IoT into modern manufacturing
By Staff Report January 9, 2025 7:10 pm IST
IoT is the backbone of modern cutting tool management systems, integrating machines, sensors, and devices through a connected network. AI-driven predictive maintenance uses AI algorithms to analyse real-time data from cutting tools. Akash Abaji Kadam, a Design Engineer at Caterpillar Inc., highlights the importance of Artificial intelligence (AI), machine learning (ML), and Internet of Things (IoT) integration in cutting tool management.
AI, ML, and IoT integration in cutting tool management
Integrating artificial intelligence, machine learning, and the Internet of Things (IoT) has assisted with managing and monitoring cutting tool systems in the manufacturing sector. These advanced technologies enable real-time precise decision-making for productivity improvement, cost reduction, and improved quality of machined components through accurate and predictive decision-making.
IoT is the backbone of modern cutting tool management systems, integrating machines, sensors, and devices through a connected network. IoT-embedded cutting tools integrate with sensors to monitor temperature, vibrations, cutting force, spindle speed, and tool wear. For example, IoT-enabled cutting tools monitor tool life and actual cutting forces in real time to prevent defects in high-value aerospace components and save time and material in aerospace manufacturing.
These sensors transmit data to a central platform that performs real-time analytics on tool performance. This enables operating staff to monitor the cutting tool’s status remotely, ensuring optimal conditions for continuing the machining process. For example, a system automatically alerts operators when a tool’s temperature exceeds its maximum limit, preventing any damage to the tool or work environment.
AI-driven predictive maintenance
AI algorithms analyse historical and real-time data from cutting tools to predict when maintenance is required. This approach, known as predictive maintenance, enables tool replacement before failure, minimising unplanned downtime. Unlike scheduled maintenance, the AI-driven predictive models optimise tool usage and reduce unnecessary replacement.
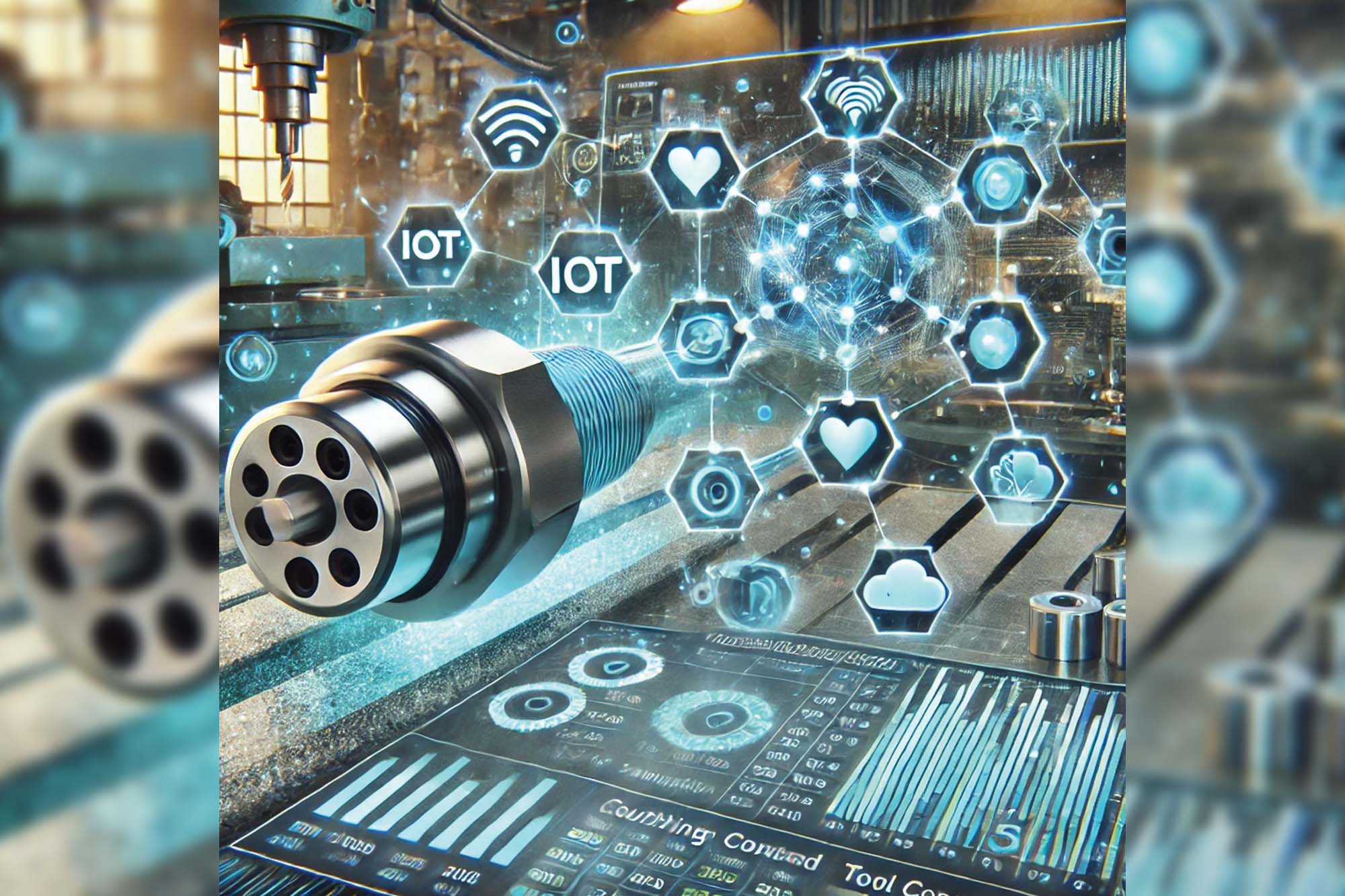
AI systems analyse IoT sensor data to identify wear patterns and predict tool lifespan with advanced algorithms, ensuring tool accuracy.
Dynamic maintenance schedules based on real-world conditions can lead to extended tool life, reduced downtime, and lower maintenance costs. Practical example – With integrated artificial intelligence, the computer numerically controlled machining centre continuously analyses tool conditions and automatically schedules replacement, ensuring no downtime on the production line.
ML-powered process optimisation
Machine Learning (ML) is the most important technology for optimising cutting tool parameters with increased efficiency and performance. ML algorithms analyse large datasets during machining to identify optimal cutting conditions, including feed rate, cutting speed, depth of cut, applications, adaptive machining, and quality control.
Adaptive machining is a machine-learning technique that adjusts real-time parameters, considering the cutting tool’s condition and material properties. Quality control algorithms detect anomalies that could impact the machining process’s surface finish or dimensional accuracy. Practical examples include automotive manufacturers implementing the ML model for finer cutting, decreased cycle times, and quality repetition during mass production.
Smart tool tracking and inventory management
Coupling IoT with AI improves inventory management and provides elaborative insights into the usability and availability of the tools. Smart systems track location, frequency of usage, and estimated remaining life for any tool to ensure availability. In precision engineering industries, automated inventory management ensures the availability of appropriate coated tooling for specific geometric cutting tools and specialised machining operations.
Digital twins for virtual monitoringWhen powered by AI and IoT systems, digital twin technology can create a cyber-replica of the cutting tool, incorporating digital models of key machining processes like tool wear patterns, thermal effects, and vibration impacts.
It has certain advantages, including real-time simulation of tool performance, proactive problem identification, and optimisation of machining strategies. A mould-making company uses digital twins to simulate high-speed milling operations, reducing trial and error in toolpath planning to improve productivity.
Integration of cloud platforms for data analytics
Cutting tools utilise IoT connectors to connect to cloud platforms for advanced analytics using AI and machine learning algorithms. Cloud computing provides manufacturers benchmark tool performance across multiple facilities, identifies long-term tool wear and failure trends, and shares real-time information with stakeholders. Practical example – Global manufacturers utilise cloud-based monitoring systems for consistent cutting tool effectiveness and consistent quality production across their international plants.
Human-machine collaboration in decision-making
While AI and IoT automate tasks, the role played by humans remains fundamental. While systems do nothing but provide pointed insights, skilled operators interpret them to make informed decisions. A practical example is that during high-mix, low-volume production, machinists collaborate with AI systems to create machining strategies for one-off components.
Sustainability and waste reduction
AI and IoT are revolutionising sustainability by optimising tool usage and reducing waste. Predictive maintenance and precise process control reduce scrap rates, while IoT monitoring keeps the tools operating within safety parameters. For example, AI-driven systems track tool efficiency in green manufacturing initiatives to reduce energy consumption and material waste.
Future outlook
Cutting tool management is rapidly evolving as it integrates AI, ML, and IoT technologies. Emerging technologies like 5G connectivity and edge computing combined with augmented reality enhance the systems by enabling 5G ultra-fast data transmission for real-time analytics, processing the data locally to reduce edge computing latency and providing AR immersive interfaces for tool monitoring and training.
As these technologies mature, they will make cutting tool management autonomous, adaptive, and efficient to standards never before seen within manufacturing.
Conclusion
Integrating the power of AI, ML, and IoT into managing and monitoring systems of cutting tools yields critical transformative benefits for a manufacturer. Allowing real-time monitoring, predictive maintenance, process optimisation, and sustainability answers the current challenges in manufacturing. As industries continue with digital transformation, AI, ML, and IoT are crucial for cutting tool applications in industries undergoing digital transformation, as they will enhance productivity and competitiveness in a dynamic market.
Cookie Consent
We use cookies to personalize your experience. By continuing to visit this website you agree to our Terms & Conditions, Privacy Policy and Cookie Policy.