Reloading smart factories with AI-ML
By Staff Report December 2, 2024 2:40 pm IST
Everything is turning smart, from phones to watches. So, the manufacturing of these smart appliances has become even smarter. Adopting artificial intelligence (AI) and machine learning (ML) in manufacturing is a step towards making these smart factories, which presents a complex mix of challenges and opportunities – digital literacy and data management to cost concerns and cultural resistance. We address some of these challenges and what opportunities could be unlocked by solving them.
We all are familiar with LG Electronics. They are leading the world, for example, with transforming their factory in Changwon, South Korea, into LG Smart Park, a futuristic hub of home appliance manufacturing. The key elements of the transformation are a digitally-enabled end-to-end three-dimensional logistics system, an advanced analytics system based on edge computing technology and machine learning that predicts defects, and state-of-the-art facilities that can mass-produce multiple models to respond flexibly to customer requirements.
Another household name, Adidas, developed Speedfactories, where automation was central to their work. Robots took the place of human labourers to boost savings and efficiency. The list includes Ford’s Michigan factory, John Deere’s farm equipment manufacturing unit in the Midwest, USA, Siemens’ digital factory, and many more. All the leading businesses have adopted advanced technologies like AI and ML within a very short span of their introduction. So, what can manufacturers expect when creating a smart factory with AI and ML?
With ML, users can detect all their problems and closely check their inventory management, assets, logistics, and supply chain management. Product development, quality control and security check-ups are other boons. Al will automate all your complex tasks and make them consistent for you. The benefits are well-known and can be browsed at any time. The real challenge manufacturers face is adopting these technologies when everyone else is around.
When we asked Amit Saluja, Founder of digital Consulting LLP, about the challenges, he shared how people need more digital literacy and they should avoid ad hoc implementation while adopting AI in smart practices. Digital literacy requires understanding long-term data availability, while ad hoc implementation requires a mindset shift to focus on building a roadmap and assessing ROI based on the right data points. Inaccurate or insufficient data points can lead to ineffective ROI assessments and hinder the adoption of AI in smart practices.
Manufacturers should understand four key aspects while adopting AI: strategy, roadmap, digital literacy training, and patience. AI and ML are a long journey, not a quick solution. Patience and adaptability to the changing landscape are requisites.
Pujan Ghelani, Director of Partnerships & Business Development, Symphony AI, shares, “Manufacturers confront hurdles when implementing AI in smart practice, including change in management and pragmatism in selecting the best solution. It matters to have a rationale and purpose for adopting technology rather than simply using it because everyone else is.”
Manufacturing plant operational costs and solutions
Over the past few years, operational costs in manufacturing plants have increased due to various factors, including increased raw material prices, workforce costs and availability, equipment ageing, and increased power consumption. These factors create a complex and costly operation in the industry.
Amit Saluja shares that the focus on logistics has increased the overall cost of a manufacturer’s supply chain, and organisations are experiencing impacts across all functions. Adopting lean principles, streamlining processes, or using technology solutions to address specific areas by focusing on them and implementing them effectively.
Building a roadmap is important to adopt digital infrastructure and AI solutions in supply chain and manufacturing operations. Solutions depend on the availability of infrastructure, which refers to the connectivity within the plant at a machine level. This ensures the data coming to the enterprise resource planning (ERP) system is accurate and not manipulated or subject to human errors. So, the initial focus is to build an infrastructure that is ready for AI and ML.
Many manufacturers are hesitant to adopt AI-based asset or predictive maintenance tools as they believe they are unreliable and can be influenced by market trends. Only 25–30% of plants are using condition monitoring and predictive maintenance, with only 1% effectively using it. This presents an opportunity hindered by a skill gap and a lack of the right data points to estimate ROI.
The manufacturing firm must decide how to reduce its price because a single product has multiple competitors. AI and ML can help here by curating the data and revealing a roadmap that can be followed. If the user decides that product cost is the problem, they can solve it, and if the problem is employee management, even that can be resolved. As shared by Akash Kadam, Design Engineer, Caterpillar Inc, Streamlining operations can reduce operational costs.
Pujan Ghelani shares an experience, “My recent conversation with an old chocolate manufacturer in Europe highlighted the need for a solution as they are bleeding money and laying off employees. They are short on time and cannot wait for results or invest heavily. Organisations need to identify and get early value for their customers to realise value. This is representative of many customers who are looking for ROI. The most effective approach is prioritising use cases and assigning resources to address problem statements by focusing on the best validation for a technology or solution, such as predictive maintenance or inventory. This approach should be based on immediate value and today’s needs.
Data Management
When discussing data management, we look for clean data, which is now manipulated through various methods. The first immediate step is to address the availability of instrumentation, which can be directly applied to equipment and processes or collected through various applications or systems.
The second point is that end customers often have data, which is stored for a different amount of time. Users can build models on data collected for a year and deploy them to build their future.
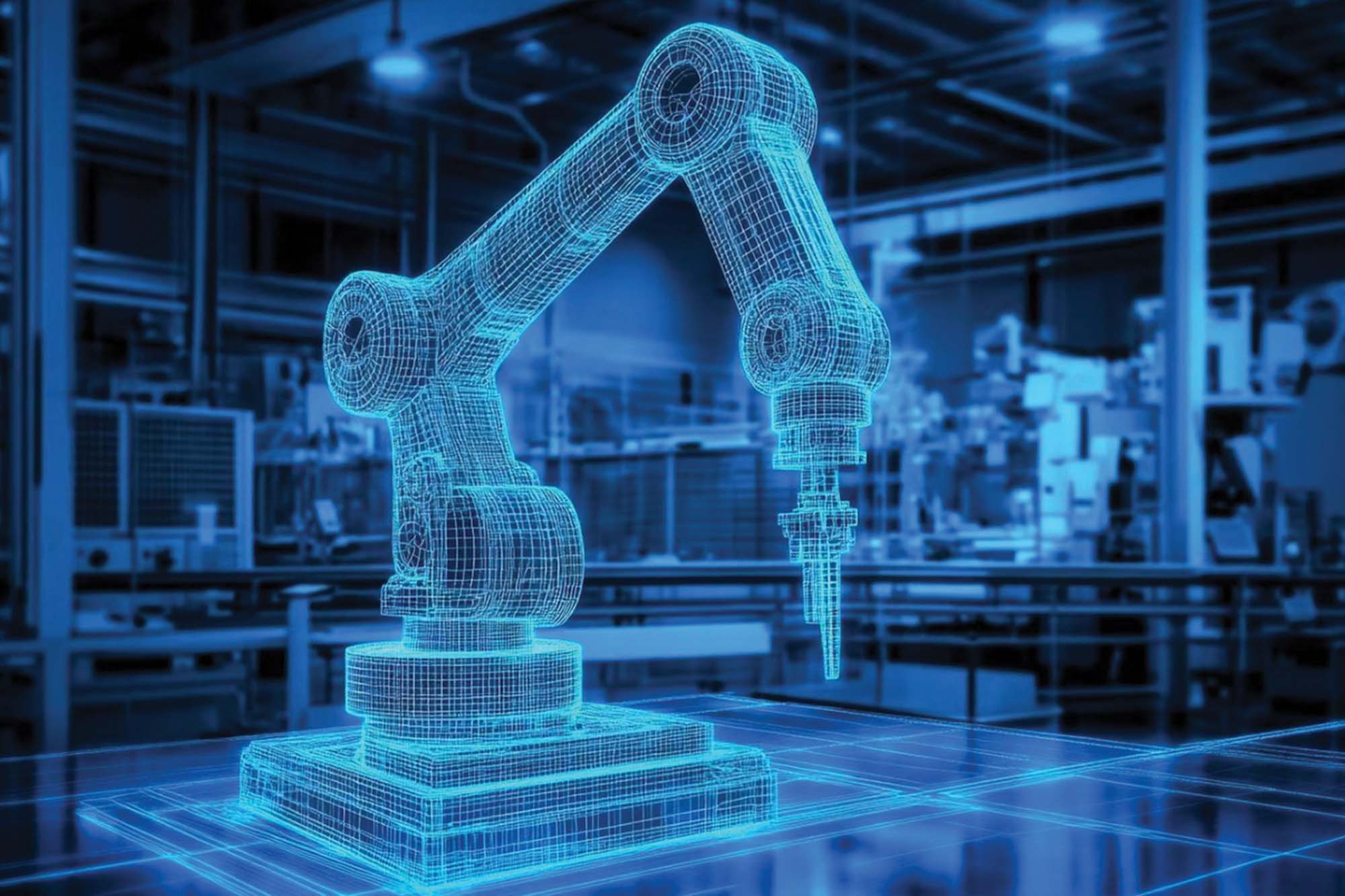
Manufacturers need to understand that AI models can automate 40–50% of work in predictive use cases like motors, but the generated alerts require human intervention to ensure proper action is taken. This is particularly important when applying AI models to motor use cases, where predictive insights are for efficient operation.
Tarun Sharma, COO of NRV DesignX Pvt Ltd, says AI can alert you when a problem arises or might arise and inform manufacturers to take action. If the model is trained for auto-adjustment, it can increase efficiency and reduce downtime by 35% to 40%.
AI ApplicationAkash Kadam says it’s a myth that AI is a technology exclusively designed for large companies. Large companies have already adapted to them and face challenges different from those faced by SMEs when adapting to change. Even students are using AI now, citing that it has become a household thing. SMEs lack digital data, which is important for AI applications. To implement AI in SMEs, they must invest in reliable digital data processes. This will make AI integration easier and reduce the need for ROI. Data quality is important for SMEs, as it determines the ROI. SMEs should focus on refining processes related to reliable digital data to make AI integration easier.
Even high-scale industries with over 1000 parts in generation face financial constraints due to the need for constant innovation and assembly. AI and ML can help this by tracking data and deploying only a few people, and the system can notify workers, control inventory, and schedule parts accordingly. Today, manufacturing shops estimate prices before submitting proposals, maintaining efficient operations and cost-effectiveness.
AI-based scheduling techniques are available to track machine performance and operation times, extract data on machine time, starting and ending times, and micromanage every machine or operation in the shop. This technique is for flawless scheduling and managing shop operations.
The estimation system relies on experience and operator input but lacks real-time tracking. AI can improve it by scheduling machines based on shipping time and the number of operations each part can take care of. This is for accurate predictions and efficient scheduling. ML will learn from previous data and history, predicting things accurately. AI can also combine multiple parts based on machine algorithms and ML data, reducing operational time and increasing competitiveness.
Smart factories are constantly evolving, and it is a journey that manufacturers can embark on early on. The smartness of any factory is the result of smart data analysis and evenly smartly managing it, making your data work for you and build for you. So, despite the challenges, minimal infrastructure changes and updates will assist in efficiently using Al and ML to become smart.
—————————
Amit Saluja, Founder, digiXLT Consulting LLP.
Rising raw material costs, skilled workers availability, pressures of improving process and machine efficiency, quality issues, managing downtimes and uncertainty in demand are creating huge challenges. Digitalisation can help address these but manufacturers are struggling with adoption due to lack of structured process. At digiXLT we help enterprises build technology roadmaps through digital maturity assessment & design thinking workshops and guide on prioritising solutions for adoption.
Mr Akash Kadam, Design Engineer, Caterpillar Inc.
AI-based techniques allow manufacturers to schedule and track machine performance and operation times, enabling micromanagement of every machine or shop operation. AI can also combine the functions of multiple parts based on machine algorithms and data.
Mr Pujan Ghelani, Director of Partnerships & Business Development, Symphony AI.
The biggest issue in data management is the availability of clean data, which can be manipulated through various methods. Addressing this issue requires the availability of instrumentation, which can be directly applied to equipment and processes or collected through various applications or systems.
Mr Tarun Sharma, COO, NRV DesignX Pvt Ltd.
AI models can automate 40–50% of work in predictive use cases like motors, but human intervention is needed to ensure proper action is taken. AI can alert manufacturers when problems arise or might arise, increasing efficiency and reducing downtime by 35–40%.
Cookie Consent
We use cookies to personalize your experience. By continuing to visit this website you agree to our Terms & Conditions, Privacy Policy and Cookie Policy.